- Generative AI Enterprise
- Posts
- 25 must-read AI accelerators
25 must-read AI accelerators
Plus, key takeaways to help you level up fast.
WELCOME, EXECUTIVES AND PROFESSIONALS.
I analyzed 2,537 AI accelerators. These 25 stand out beyond the hype.
Executive playbooks, case studies, best practices and market insights packed with value on gen AI, agentic AI, and AI transformation.
BEST PRACTICE INSIGHT & CASE STUDIES

Image source: Boston Consulting Group
Brief: Boston Consulting Group (BCG) released eight playbooks to guide CEOs and senior executives (CFOs, COOs etc.) on AI transformation, including gen AI. Each ~25-slide playbook draws from 1,000+ AI programs, offering strategies and case studies to drive enterprise value.
Breakdown:
For Finance Leaders, the 23-slide Finance playbook explores AI opportunities, evolution of processes, operating models, and more.
For Technology Leaders, the 24-slide Data & Digital Platforms playbook covers maximizing data value, evolving tech stacks for AI, and more.
For Operations Leaders, the 20-slide Supply Chain playbook highlights gen AI applications and starting points, while the 24-slide Customer Service playbook explores economics, teams, and more.
For Risk Leaders, the 32-slide Risk & Compliance playbook details AI-driven risk management, capability evolution, and more.
For Sales Leaders, the 23-slide Customer Engagement playbook covers ideation, personalization, and communication, while the 23-slide B2B Sales playbook focuses on team evolution, strategies, and more.
For People Leaders, the 22-slide HR playbook outlines future structures, tools, skills, and gen AI performance gains.
Why it’s important: These playbooks for leaders provide actionable strategies and learnings to increase the likelihood of success in realizing enterprise value from AI investments.
Full report here. Date published: July - December 2024
BEST PRACTICE INSIGHT & CASE STUDIES

Image source: MIT
Brief: MIT’s report Leading With AI explores how enterprises are leveraging gen AI, featuring insights and real-world case studies from eight companies. Each case is presented as a graphic detailing approaches and value delivered.
Breakdown:
Case studies include Pfizer speeding up knowledge transfer, Comcast improving customer call responses, and Takeda accelerating drug design.
It also features Dicks Sporting Goods creating personalized email campaigns faster, along with four case studies.
It also features Accenture redesigning their workforce for gen AI and McKinsey improving document processing, along with four other case studies.
Scaling AI or gen AI is a top priority for 82% of C-suite leaders, while 80% of chief data officers believe gen AI will transform their business.
AI has been shown to improve worker performance by up to 40% when used for appropriate tasks.
Leaders should focus on solving critical business problems with AI, ensuring their data is AI-ready, and addressing skills gaps among employees.
Why it’s important: MIT provides leaders with actionable insights and detailed real-world case studies to help integrate gen AI effectively, drive innovation and enhance workforce performance.
Full report here. Date published: October 2024
BEST PRACTICE INSIGHT

Image source: Deloitte
Brief: Deloitte published its AI Governance Roadmap, a 16-slide guide, to help boards understand their role in AI governance. It explores key questions and resources for overseeing AI, regardless of the organization's AI maturity.
Breakdown:
Evaluate AI approach within corporate strategy, oversee execution, and help management adapt strategy to AI risks and opportunities as needed.
Oversee AI risks (strategic, functional, and external) to the company’s overall strategy, integrating them into the enterprise risk program.
Define oversight ownership at the board level (e.g. the full board, existing committee, new committee, or sub-committee). Consider an AI advisory council or adding an AI-expert board member.
Monitor performance against AI-specific strategic, financial, and operational goals. Establish a consistent evaluation process.
Assess if management has the skills to execute the AI strategy. Understand AI’s impact on recruitment, development, and incentives.
Cultivate trustworthy AI with appropriate disclosures and communications. The board tracks AI usage, ensuring adherence to ethical standards.
Why it’s important: Although AI is not new, the increasingly proliferation of gen AI in enterprises brings governance topics to the forefront. The decisions leaders make today in balancing opportunity and risk will shape their enterprises and society at large for years to come.
Full report here. Date published: February 2025
VALUE ASSESSMENT

Image source: KPMG
Brief: To help clients accelerate AI transformations, KPMG spent 18 months analyzing 3 billion+ data points for 17 million+ companies worldwide to quantify the value at stake from fully deploying and adopting gen AI.
Breakdown:
The gen AI opportunity equates to 4-18% of EBITDA or 19-23% of salary cost annually in labor productivity alone across sectors.
Productivity gains were quantified by estimating freed-up time, multiplied by salaries, assuming reinvested time is equally productive.
48% stems from low/medium complexity tasks, while 52% involves high-complexity work requiring tailored solutions, agents and complex change.
EBITDA impact varies by sector, with the highest in Professional Services (17.6%), Technology, Media & Telecom (15.5%), and Healthcare (14.6%).
Front Office (excl. Sales & Marketing) holds 22.4% of gen AI’s value. IT (16%), Sales (15.8%), Supply Chain (15.4%), Finance (9.3%), and Marketing (6.4%).
Why it’s important: Executives remain willing to invest in gen AI for productivity and competitive advantage, but often face challenges in quantifying expected returns and setting realistic targets. KPMG’s value assessment helps address these challenges, shaping strategy, guiding investments and accelerating transformation.
Full report here. Data published: March 2025
MARKET INSIGHT

Image source: Harvard Business School
Brief: Harvard Business School conducted a study with 776 professionals at consumer goods giant Procter & Gamble (P&G) to assess AI’s impact on individuals and teams in developing products and retail strategies.
Breakdown:
Individuals randomly assigned to use AI, GPT-4 and GPT-4o in this study, performed as well as a team of two without AI.
Teams using AI were far more likely to produce top-tier solutions, ranking in the top 10% for quality, as shown in the graph above.
Both AI-enabled individuals and teams worked faster, saving 12-16% of the time spent by those not using AI.
AI broke down silos, enabling R&D teams to be more business-driven and commercial teams to create more technical solutions.
Teams using AI were happier, reporting higher energy and enthusiasm, while also experiencing less anxiety and frustration than non-AI users.
Why it’s important: In recent years, much of the focus has been on productivity of individual knowledge workers like consultants, lawyers, and coders. But a lot of work happens in teams, which offer benefits individuals alone can't typically provide, such as better performance and satisfaction.
Full report here. Date published: March 2025
BEST PRACTICE INSIGHT & CASE STUDIES

Image source: Google
Brief: Google’s 76-page “Agents Companion” paper builds on its original “Agents” paper, exploring the operationalization of gen AI agents. It covers AgentOps, evaluation, Agentic RAG, multi-agent systems, real-world case studies, and more.
Breakdown:
In operationalizing agents, Google emphasizes the importance of metrics within AgentOps to help build, monitor, and compare agent revisions.
For agent evaluation, it focuses on assessing agent capabilities, evaluating trajectory and tool use, and evaluating the final response.
AI is evolving towards multi-agent systems, specialized agents working together to achieve complex goals. The paper outlines design patterns.
Agentic RAG architecture is highlighted: autonomous retrieval agents that actively refine their search based on iterative reasoning.
Google showcases how specialized agents collaborate to power in-car conversational AI, illustrating real-world multi-agent systems in action.
Why it’s important: Gen AI agents mark a leap beyond standalone LLMs, enabling dynamic problem-solving and interaction. This “102” guide builds on core concepts, offering in-depth exploration of agent evaluation methods and practical applications to help enterprises operationalize results in production.
Full report here. Date published: March 2025
MARKET & BEST PRACTICE INSIGHT

Image source: McKinsey & Company
Brief: McKinsey published its 2025 state of AI report, surveying 1,491 survey participants across 101 nations, exploring AI adoption, how organizations are capturing value, and perspectives from McKinsey leaders.
Breakdown:
Fifty-three percent of surveyed executives say they are regularly using gen AI at work, compared with 44 percent of midlevel managers.
The survey highlights AI deployment structures: 57% centralize risk and compliance, while tech talent and AI adoption often follow a hybrid model.
27% of orgs using gen AI review all AI-generated outputs, while a similar share says 20% or less is checked before use.
Of gen AI best practices, tracking well-defined KPIs drives the most impact. At larger orgs, a clear adoption roadmap is also among the most effective.
AI hiring remains tough, though fewer respondents than in past years say filling roles is “difficult” or “very difficult.”
Inaccuracy, cybersecurity, and IP infringement are the top gen-AI risks causing negative impacts, according to survey responses.
Why it’s important: Gen AI use continues to surge, but it’s still early days. While enterprises are increasingly realizing value, more than 80 percent of respondents say their organizations aren’t currently seeing a tangible impact on enterprise earnings from their use of gen AI.
Full report here. Date published: March 2025
BEST PRACTICE INSIGHT & CASE STUDIES

Image source: IBM
Brief: IBM published a 48-page report on what AI transformation success looks like across 10 industries, featuring enterprise case studies, executive perspectives, and recommended actions for each industry.
Breakdown:
Enterprises fixated on efficiency risk missing AI’s true potential: innovation, growth, and breakthroughs once thought not possible.
Achieving leadership requires an operating model that is people-centered, process-driven, technology/data-enabled, and partner-energized.
The report details what AI transformation success looks like across Finance, Retail, Telecom, Automotive, Healthcare, and other industries.
It features case studies and perspectives from NatWest, Bharti Airtel, NASA, Honda, the UK NHS, Informed Genomics, and more.
For instance, NatWest’s Cora+ AI, leveraging RAG, addresses over 11 million customer inquiries annually, improving satisfaction by 150% through more nuanced, anticipatory responses.
Wendy Renshaw, Chief Digital Officer at NatWest, emphasizes the importance of customer care, whether through human or digital interactions.
Why it’s important: Most CEOs recognize that leaders in gen AI, who go beyond productivity to drive growth, will capture greater competitive advantage. Successfully leveraging AI will be a defining factor in shaping the rise, or fall, of businesses across virtually all industries.
Full report here. Date published: February 2025
CASE STUDY

Image source: McKinsey & Company
Brief: A new McKinsey case study reveals how its transformed work with its gen AI platform, Lilli. The objective was to build a platform powered by its proprietary knowledge to accelerate and improve insights for its teams and clients.
Breakdown:
Proof of Concept (March 2023, 1 week): A small team built a lean prototype in 1 week and secured investment approval.
Roadmap & Operating Model (April 2023, 2 weeks): Aligned on priority use cases based on value, impact, feasibility and requirements. Set up cross-functional agile squads for delivery.
Development Decisions (May 2023, 2 weeks): Guided by a five-point framework that evaluated cost, scalability, performance, security, and timing. Combined a hyperscaler’s prebuilt model with five of its own smaller expert models for enhanced answer relevance.
Build, Test & Iterate (May 2023, build: 5 weeks, test: 3 weeks): Alpha tested with 200 users, with rapid feedback improving response quality.
Firmwide Rollout (July 2023, 3 months): Gradual rollout over 3 months, available to all employees by October 2023.
The platform helps employees quickly learn new topics, access McKinsey frameworks, analyze data, develop presentations in McKinsey's style, create project plan drafts, and more.
72% of employees are active on the platform, saving up to 30% of time and processing over 500,000 prompts monthly.
Why it’s important: McKinsey's swift development of Lilli demonstrates how enterprises can leverage gen AI to accelerate knowledge work and enhance productivity. McKinsey's full case study also details lessons learned and further insights into the platform.
Full case study here. Date published: November 2024
BEST PRACTICE INSIGHT

Image source: Anthropic
Brief: Anthropic published an article sharing best practices from building effective agents with teams across industries, identifying seven common agentic system patterns in production and when to use them.
Breakdown:
Anthropic distinguishes between two types of agentic systems: workflows, where LLMs and tools follow predefined code paths, and agents, where LLMs dynamically direct their own processes and tool usage, controlling task execution.
Anthropic is seeing seven common agentic system patterns in production: Augmented LLM (building block), prompt chaining, routing, parallelization, orchestrator-workers, evaluator-optimizer (all workflows), and agents.
For instance, in the orchestrator-workers workflow, a central LLM divides tasks, assigns them to worker LLMs, and combines their results, making it ideal for tasks like complex coding and data searches across multiple sources.
Agents are emerging as LLMs advance in understanding complex inputs, reasoning, planning, tools, and error recovery. Ideal for open-ended problems where the steps are unpredictable and a fixed path can't be hardcoded.
For full details on all seven patterns, including architecture diagrams, usage guidance, and implementation examples, see the summary or full article.
The full articles also dives into customer support and coding agents, which have shown particular promise, as well as prompt engineering best practices and the use of frameworks like LangChain (see Anthropic’s cookbook).
Why it’s important: Anthropic's experience in building agents offers best practices to help enterprises leverage gen AI. These patterns can be adapted and combined for various use cases. While more agentic complexity can improve performance, it often increases latency and cost, so such tradeoffs should be considered.
Full report here. Date published: December 2024
ENTERPRISE TRANSFORMATION
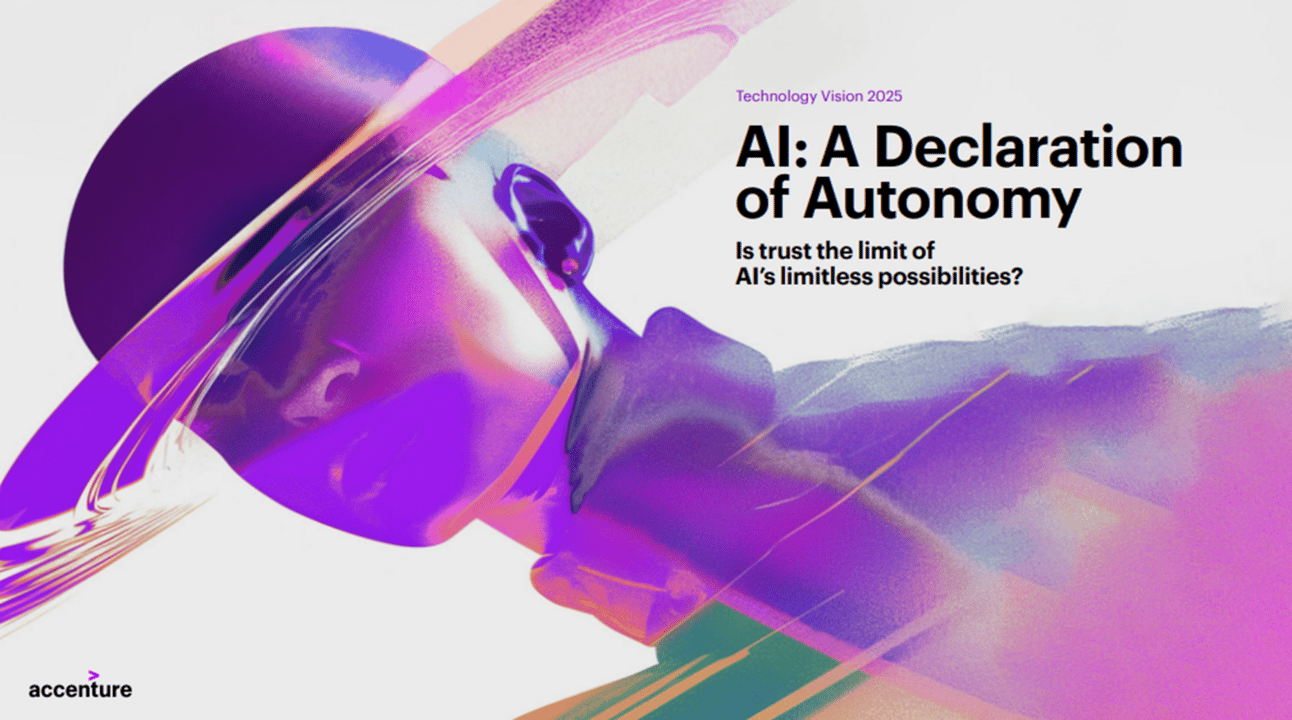
Image source: Accenture
Brief: Accenture’s 67-slide report, published by CTO Karthik Narain and his research team in January 2025, envisions a profoundly different, autonomous workplace reshaped by gen AI agents, and explores what it takes to build this future.
Breakdown:
As gen AI becomes central to enterprise tech, development costs drop and new systems emerge. 77% of execs agree agents will reinvent systems.
AI agents can personalize at scale but risk brand homogenization. 80% of leaders worry chatbots sound generic, challenging differentiation efforts.
Robots with embedded LLMs have generalist versatility, moving past pre-programmed limits. 74% of leaders see the promise of intelligent robots.
Gen AI empowers employees by amplifying their capabilities, creating a feedback loop that expands the autonomy of people and AI over time.
Leaders see the challenges of creating this future, including high upfront investments, data quality, new skills, and most importantly, building trust.
Why it’s important: Leaders must prepare for a world where AI is everywhere and acting autonomously on behalf of people. Forward-thinking enterprises are already leveraging this transitional period to invest in building the trust needed for innovation, growth and making this future a reality.
Full report here. Date published: January 2025
BEST PRACTICE INSIGHT & CASE STUDIES

Image source: Microsoft
Brief: Microsoft published The AI Decision Brief, a 38-slide guide for AI leaders on navigating gen AI. It covers the landscape, future potential, and best practice strategies, featuring executive perspectives, market studies, and real-world examples.
Breakdown:
AI capabilities are accelerating rapidly, doubling 4x faster than historical progress in client-server computing, the internet, and mobile/cloud.
AI usage and sentiment is trending upwards with leading “AI-rich” enterprises achieving 4.9x average ROI.
Microsoft emphasizes learning from AI-native startups, software firms, co-innovation labs, and research groups to help drive innovation.
Best practices in areas like strategy and data are highlighted as enterprises progress from exploring AI to scaling it for maximum value.
Brad Smith, Vice Chair and President, and Nathalie D’Hers, CVP Microsoft Digital, are among the many executives who share their perspectives.
Dentsu’s success in cutting time to media insights by 90% using Azure AI and Copilot is shared, alongside other enterprise case studies.
Why it’s important: Microsoft holds a strong position in the enterprise AI market, with 70% of Fortune 500 companies using Microsoft Copilot, its OpenAI partnership, and Azure's 25% share of the global cloud market. Leveraging experiences from its executives and customers can help turn AI ambitions into lasting value.
Full report here. Date published: February 2025
PLATFORM TRANSFORMATION

Image source: Goldman Sachs
Brief: Goldman Sachs published insights on AI’s role in re-platforming the economy, transforming how software and technology operates to drive value through three key vectors: compute, software, and data.
Breakdown:
Compute has progressed from traditional CPUs to powerful GPUs, with players like Nvidia, while newer CPUs are also emerging for specific AI workloads.
Software has evolved from application-centric to data-centric design, making data a foundational input for application development.
VP Ashraf stated: “These shifts have produced a '3D chessboard,' with companies from vastly different backgrounds competing for leadership.”
Major tech shifts typically see financial gains first captured by semiconductor and hardware companies, then “blossom” in the infrastructure software layer.
Infrastructure software (e.g. NVIDIA CUDA, Microsoft Fabric, Databricks) is central to AI re-platforming, linking hardware innovation at the bottom of the stack to application development at the top.
As in previous eras, infrastructure software will be the next investment frontier following outsized CapEx in AI hardware (e.g. GPUs).
Why it’s important: Enterprises who seize the platform shift early will lead in redefining existing markets and creating new ones. Goldman expects smaller AI companies to consolidate with larger players, enterprises to strengthen through M&A, and investors to increasingly focus on higher layers of the tech stack.
Full report here. Date published: March 2025
BEST PRACTICE INSIGHT

Image source: Menlo Ventures
Brief: Menlo Ventures article AI Agents: A New Architecture for Enterprise Automation explores six gen AI examples from RAG to Autonomous Agents, detailing reference architectures and levels of autonomy. Architecture summary here.
Breakdown:
The fully autonomous agents of tomorrow might possess all four building blocks of AI agents: reasoning, external memory, execution, planning. But today’s LLM apps and agents do not.
The popular RAG architecture isn’t agentic but relies on reasoning and external memory. The key distinction is that these apps use the LLMs as a "tool" for search, synthesis, or generation, but their steps remain pre-determined by code.
By contrast, agents emerge when the LLM controls the application's flow, dynamically deciding actions to take, tools to use, and how to interpret and respond to inputs. Menlo ventures outlines three types of agents.
Decisioning Agent: At the most constrained end are “decisioning agent” designs, which use LLMs to traverse predefined decision trees.
Agent on Rails: “Agents on rails” offer more freedom with a higher-level objective, but constrain the solution space with an standard operating procedure (SOP) and a predetermined library of tools to choose from.
General AI Agents: At the far end of the spectrum are “general AI agents”, essentially for-loops with minimal data scaffolding, relying entirely on the LLMs reasoning for planning, reflection, and course correction.
Why it’s important: Gen AI is entering its agents era, marking a shift toward greater autonomy and sophistication in AI systems. To fully leverage their potential, it’s key to understand agentic designs and the nuances of varying definitions emerging across the market.
Full report here. Date published: September 2024
MARKET INSIGHT

Image source: Anthropic
Brief: Anthropic launched The Anthropic Economic Index revealing “the clearest picture yet” of how AI is being used in real-world work. The results, detailed in a 38-page report, show AI is primarily used to augment human capabilities, among other findings.
Breakdown:
Despite many surveys on AI’s impact on work, large-scale empirical evidence on how these systems are actually used for different tasks remains scarce.
Anthropic analyzed 4M+ Claude conversations, mapping tasks and occupations using the U.S. Department of Labor’s O*NET Database.
AI adoption is highest in software development and writing, accounting for nearly half of total use. Adoption remains early, with ~36% of occupations using AI for at least 25% of tasks.
AI is used 57% for augmentation (e.g., learning, iterating) and 43% for automation (e.g., fulfilling requests).
The true augmentation rate may be higher: e.g. a user asks Claude to draft a memo but later refines it themselves.
Anthropic says it mitigated the limitation of analyzing only Claude Free and Pro data (excluding API and Enterprise) by filtering out non-work conversations.
Why it’s important: Many AI adoption surveys exist from advisory, research, and tech firms, but Anthropic’s stands out for its scale and system-driven, empirical approach, revealing early signals of AI’s impact as it automates, augments, and expands, shaping the future of work and transformation.
Full report here. Date published: February 2025
CASE STUDY

Image source: Meta
Brief: Meta shared an 8-page case study on how Sevilla FC used Llama to build Scout Advisor, a gen AI solution that transformed searching and analyzing over 300,000 scouting reports into a fast, accurate conversational experience.
Breakdown:
Sevilla’s in-house tools excelled at structured data but struggled with unstructured data, including valuable expert opinions.
They used Llama 3.1 70B Instruct and IBM's watsonx to create Scout Advisor, providing conversational search and player summaries.
Prompt enrichment ensures idiomatic user questions have enough context for a thorough semantic search of Sevilla FC’s 300,000+ scouting reports.
For instance, “show me talented wings” is enriched to note that a talented winger "takes on defenders with dribbling, creating space and penetrating opposition."
Instead of reviewing "45 reports to know what my scouts think," Sporting Directors get natural responses with player performance in seconds.
Plus, open-source Llama enables Sevilla to customize and optimize models without constraints from third-party APIs, securely within its environment.
Why it’s important: With 48% of enterprises planning to leverage open-source in their AI implementations in 2025, this case study highlights how prompt enrichment and semantic search, common gen AI use cases, can drive meaningful value extracting and summarizing unstructured data.
Full report here. Date published: February 2025
MARKET INSIGHT

Image source: IoT Analytics
Brief: IoT Analytics released a 263-page report on top gen AI firms, drawing from 530+ projects. The analysis covers three enterprise segments: data center GPUs, foundation models and model management platforms, and gen AI services.
Breakdown:
In just two years, the enterprise gen AI market across the three segments assessed has surged from $191M in 2022 to $25.6B in 2024.
The AI hardware market, specifically data center GPUs, experienced vast growth climbing from $17B in 2022 to $125B in 2024.
Data center GPU demand doubled in 2024. NVIDIA holds 92% market share, AMD grows from 3% to 4%, Huawei has 2%, followed by Cerebras and Groq.
Microsoft leads in “foundation models and model management platforms” with 39% market share in 2024, followed by AWS (19%), Google (15%), and OpenAI (9%).
This excludes the market for monetized consumer and enterprise generative AI apps, like ChatGPT.
The services market is more fragmented. Accenture leads with 7% share, followed by Deloitte at 3% and IBM at 2%.
Why it’s important: There is still a lot of movement to be had in the gen AI enterprise landscape. Microsoft CEO Satya Nadella recently warned the current AI hype may mirror the 1999 Dot-Com bubble. But ultimately, winners will emerge: those who consistently deliver value beyond hype and proof of concepts.
Full report here. Date published: March 2025
BEST PRACTICE INSIGHT

Image source: McKinsey & Company
Brief: McKinsey published a blueprint to help financial services leaders unlock enterprise-wide AI value. While tailored for banks, the insights are widely applicable.
Breakdown:
Typically 70–80% of incremental AI transformation value in banks comes from just 10 of approximately 25 subdomains, such as customer underwriting.
These priority areas can be transformed using a mix of gen AI, traditional analytics, and digital tools and platforms.
Integrated via a tech stack powered by multi-agent systems, incorporating 'New elements' enabled by gen AI (see exhibit 4 in full article).
New elements include multimodal conversational experiences, digital twins for simulating behavior, AI orchestration for complex workflows, AI agents for specialized tasks like fraud detection, and more.
A high-level transformation checklist emphasizes establishing a comprehensive AI vision, leveraging a range of technologies, reimagining business domains, and ensuring component reusability.
Only a few leading banks currently achieve material AI transformation value, but others have a window of opportunity to catch up in the next few years.
Why it’s important: McKinsey's blueprint outlines a path to harness AI, focusing on key business subdomains, a full-stack approach, and technologies like multi-agent systems for enterprise-wide transformation.
Full report here. Date published: December 2024
CASE STUDIES

Image source: Boston Consulting Group
Brief: A 27-slide publication from BCG offers 42 examples and nine deep drives on how leading enterprises (those scaling AI, per BCG's Build for the Future 2024 Global Study) are generating value with AI, including gen AI.
Breakdown:
The publication showcases 42 examples with measurable outcomes across nine functions: sales, customer service, pricing & revenue management, marketing, manufacturing, field forces, R&D, technology, and business operations.
For instance, a biopharma company used AI in R&D to accelerate drug discovery, achieving a 25% cycle time reduction, $25M in cost savings, and $50M–$150M in revenue uplift.
Nine detailed case studies showcase AI (including gen AI) transformation and impact across functions, such as a gen AI Co-pilot for relationship managers at a universal bank (sales).
Additional deep dives highlight AI for BPO call agents (customer service), gen AI for data governance at a payments provider (technology), AI transforming credit processing at a European bank (business operations), and more.
It explores how AI leaders (26% of enterprises successfully scaling AI value) reshape functions rather than merely deploying or inventing, along with other success factors.
Why it’s important: This publication articulates the transformative value of AI across a breadth of functions, offering deep dive examples and measurable outcomes, all presented in a clear, easily digestible format.
Full report here. Date published: December 2024
WORKFORCE EVOLUTION

Image source: Cognizant
Brief: Cognizant’s 25-page report addresses the trillion-dollar question: what to do with the workforce in the age of gen AI. Analyzing 1,000 professions, it provides leaders with an initial framework to rethink roles and realign workforce strategies.
Breakdown:
Cognizant forecasts gen AI will improve US productivity by $1 trillion annually by 2032, impacting 90% of jobs, with 52% significantly affected.
Gen AI will reshape the talent pyramid by narrowing the base (entry-level roles), which are more automatable, while expanding higher levels.
Roles that remain fundamentally unchanged by AI, such as physical highly specialized work like electricians, will experience minimal impact.
Those augmented by AI, such as executives and lawyers, will be impacted moderately. They should upskill in new ways of working with gen AI tools.
Others transformed by AI, such as programmers and financial analysts, will experience significant reskilling and evolution in their roles.
Fully automated roles, like data entry and many customer service positions, will be reassigned or phased out entirely.
Why it’s important: Enhanced, augmented, transformed, and fully automated roles exist across enterprises. Talent management decisions today are key for preparing individuals, shaping the workforce to balance automation with human expertise, and ensuring long-term success in the market.
Full report here. Date published: February 2025
MARKET INSIGHT

Image source: Menlo Ventures
Brief: Menlo Ventures surveyed 600 U.S. leaders for its 2024 Generative AI in the Enterprise report, published in November 2024, highlighting key trends in enterprise gen AI spending, adoption, and technology evolution.
Breakdown:
Enterprise gen AI spending surged to $13.8 billion in 2024, a 6x increase from $2.3 billion in 2023. Healthcare leads enterprise spending with $500 million, followed by legal ($350 million) and financial services ($100 million).
Top use case adoption includes code generation (51%), support chatbots (31%), and enterprise search (28%). Price of tools is a minor concern (1%), with leaders prioritizing tools with potential to deliver long-term value (30%).
OpenAI’s enterprise market share fell from 50% to 34%, while Anthropic doubled from 12% to 24%, and Google also gained traction. Enterprises now deploy three or more foundation models. Closed-source options hold 81% market share.
RAG adoption surged to 51% in 2024, up from 31% in 2023, while only 9% of production models are fine-tuned. Agentic architectures debuted with 12% adoption, poised to disrupt the $10 trillion U.S. services economy.
Enterprises are converging on core runtime architectures for production apps, structured across four layers: (1) compute and foundation, (2) data, (3) orchestration, and (4) tooling (detailed breakdown in full article).
Vertical gen AI apps are gaining momentum. Initial applications focused on horizontal solutions like text and image generation, but domain-specific apps are gaining traction as enterprises seek differentiation.
Why it’s important: GenAI is set to reshape the enterprise. Spending growth, verticalization, and emerging architectures signal change ahead. Enterprises that adapt quickly to these shifts could capture outsized value.
Full report here. Date published: November 2024
BEST PRACTICE INSIGHT

Image source: McKinsey & Company
Brief: McKinsey’s article explores five roles for AI in transforming strategy: researcher, interpreter, thought partner, simulator, and communicator. These roles play a part in various phases of strategy development, from design to execution.
Breakdown:
Researcher. AI summarizes and connects data across sources, such as identifying potential M&A targets by spotting under-the-radar assets.
Interpreter. AI transforms data into insights and tracks trends to advance goals, for instance by conducting "growth scans" or monitoring competitor signals.
Thought partner. AI acts as a brainstorming partner, generating ideas, challenging strategies, and uncovering blind spots to refine decision-making.
Simulator. AI enhances scenario analysis by modeling the impact of macroeconomic economics, competitor moves, and stakeholder reactions.
Communicator. AI tailors narratives for different audiences and formats, from briefs to podcasts, to help drive action.
McKinsey expands on these roles in the context of a Southeast Asian bank pursuing expansion and outlines steps to level up your strategy with AI.
Why it’s important: McKinsey believes AI can’t, and won’t, replace human logic and interpretation in complex domains like strategy. However, it can provide faster, more objective answers to improve decision-making. By improving efficiency and allowing room for creativity, AI enables bold moves needed to beat the market.
Full report here. Date published: February 2025
PLATFORM STRATEGY

Image source: Bain & Company
Brief: Bain & Company Partner Chuck Whitten explores whether agentic AI will eliminate or enhance SaaS. History, he argues, shows technological revolutions tend to expand ecosystems rather than replacing them outright.
Breakdown:
Leaders like Satya Nadella suggest agentic AI could upend the SaaS model entirely. Others believe AI will simply enhance SaaS.
Technology revolutions are rarely binary: client/server computing didn’t completely eliminate mainframes, neither did cloud with on-prem systems. Instead, they transformed and coexisted.
If AI replaces the “business logic” layer of SaaS, agentic tool builders still face a steep challenge: mastering the nuanced use cases SaaS refined for years.
To succeed, they need to establish trust and transparency with customers. Businesses won’t adopt tools they can’t control or verify, and adapting AI to highly specific workflows is nontrivial.
SaaS vendors may embed AI deeply, creating hybrid solutions that merge AI agents’ intelligence with specialized SaaS strengths.
Many incumbents’ technical roadmaps aim for this, but it demands a willingness to disrupt their own businesses from within.
Why it’s important: SaaS disruption could redefine enterprise tech, partnerships, and ultimately those who lead the market. Bain’s Whitten predicts convergence, not collapse, where AI and SaaS both complement and compete. The lesson from history: Transitions expand ecosystems rather than replace them outright.
Full report here. Date published: January 2025
CASE STUDY

Image source: LinkedIn
Brief: LinkedIn detailed how it built its AI Hiring Assistant with EON (Economic Opportunity Network), a set of custom models that improve candidate-role matching accuracy and efficiency.
Breakdown:
EON's custom Meta Llama models were trained on 200M tokens from LinkedIn's Economic Graph, including member and company data.
Reinforcement Learning with Human Feedback (RLHF) and Direct Preference Optimization (DPO) techniques were used for safety alignment.
LinkedIn adapted foundation models like Llama and Mistral, evaluating them on open-source and LinkedIn benchmarks (see image above).
LinkedIn found EON-8B (based on Llama 3.1) to be 75x cheaper than GPT-4, 6x cheaper than GPT-4o, and 30% more accurate than Llama-3.
LinkedIn is now enhancing its EON models with planning and reasoning capabilities to enable more personalized, agentic interactions.
Why it’s important: To capture ROI, enterprises are increasingly exploring the cost and customization benefits of open-source models. LinkedIn's EON showcases how in-house gen AI innovation with domain-adapted foundation models can improve the recruiter-candidate experience while reducing costs.
Full report here. Date published: December 2024
INDUSTRY INSIGHT & CASE STUDIES

Image source: World Economic Forum
Brief: The World Economic Forum (WEF) published nine white papers on AI transformation across industries and regions, written in collaboration with Accenture, BCG, McKinsey, and the University of Oxford.
Breakdown:
The 30-page Cross-Industry AI in Action paper explores AI opportunities, adoption trends, its future potential, and features 19 case studies.
The 26-page Rise of AI Agents paper examines virtual and embodied AI, The 28-page Cybersecurity paper covers AI risks and mitigation strategies.
The 29-page Energy paper details scaling sustainable AI across nine industries. The 27-page Financial Services paper highlights AI value creation and risks.
The 30-page Healthcare paper explores AI visions and scaling challenges. The 33-page Transport paper analyzes AI's role in cutting emissions by 15%.
The 28-page Media paper covers trends and adoption. The 32-page China paper highlights AI-led industrial growth and policy collaboration.
Why it’s important: This series offers a comprehensive mix of analysis into specific industries and regions. It provides actionable guidance for organizations navigating AI transformation, emphasizing responsible practices to unlock AI’s full potential while ensuring sustainable innovation.
Full report here. Date published: January 2025
MARKET INSIGHT

Image source: Sequoia
Brief: Sequoia, the prominent Silicon Valley venture capital firm, discusses in its article Generative AI’s Act o1 how startups beating corporate IT and global systems integrators by focusing on gen AI at the application layer is "pretty doable".
Breakdown:
Competition in the foundational layer (infrastructure and models) is stabilizing, led by Microsoft/OpenAI, AWS/Anthropic, Meta, and Google/DeepMind, due to high capital requirements.
GenAI is evolving from "thinking fast" (pre-trained responses) to "thinking slow" (reasoning at inference), unlocking the potential for disruptive "killer apps" in consumer and enterprise markets.
The cloud transition brought software-as-a-service. The AI transition is about building applications that automate and amplify knowledge work, 'service-as-software', a much larger market worth trillions.
However, transforming gen AI capabilities into reliable end-to-end enterprise applications still requires significant expertise and engineering effort.
Established enterprises must adapt or risk being outpaced by new businesses that master the intricacies of knowledge work and manage to reach customers.
Similar to the emergence of 20 $1 billion+ companies in cloud and mobile applications, Sequoia expects a comparable opportunity with AI.
Why it’s important: There is vast potential for enterprise disruption and reinvention. Innovation at the application layer will play a key role in defining the next generation of industry.
Full report here. Date published: October 2024
BEST PRACTICE INSIGHT & CASE STUDIES

Image source: Galileo
Brief: Galileo, a company that specializes in AI evaluation, released a 93-page guide on mastering AI agents. It covers agent capabilities, real-world use cases, and frameworks, with a strong focus on performance evaluation.
Breakdown:
Chapter 1 introduces AI agents, their ideal uses, and scenarios where they can be excessive. It includes real-world cases from Salesforce and Oracle Health.
Chapter 2 details frameworks: LangGraph, Autogen, and CrewAI, providing selection criteria and case studies of companies using each.
Chapter 3 explores how to evaluate an AI agent through a step-by-step example using a finance research agent.
Chapter 4 covers measuring agent performance across systems, task completion, quality control, and tool interaction, with five detailed use cases.
Chapter 5 addresses why many AI agents fail and provides practical solutions for successful AI deployment.
Why it’s important: As AI agents become more prevalent, ensuring they work correctly and safely is key. This is where evaluation comes in. Galileo’s previous guide focused on "Mastering RAG," building enterprise-grade systems. Now, they’ve taken it further with agents using LLMs to complete broader, more complex tasks.
Full report here. Date published: January 2025
MARKET INSIGHT

Image source: McKinsey & Company
Brief: McKinsey’s 47-page report finds the biggest barrier to scaling AI is not employees, but leaders who aren't steering quickly enough. Inspired by Reid Hoffman’s new book 'Superagency,' it explores amplifying human potential with AI.
Breakdown:
Employees are more ready for change than leaders imagine. 3x more employees use gen AI for a third or more of their work than leaders think. 70% believe it’ll change 30% or more of their work within 2 years.
Companies need to move fast. Employees trust leaders to balance speed with safety. 47% of C-suite say gen AI is moving too slowly, despite 69% having invested over a year ago.
Employees are 1.3x more likely to trust their own companies to deploy gen AI right than other institutions.
Almost all companies invest in AI, but just 1 percent believe they are at maturity. 92% plan to invest more over the next 3 years.
Leaders need to recognize their role in driving gen AI transformation. Executives are 2.4x more likely to cite employee readiness as a barrier than leadership.
48% of employees say training is key for gen AI adoption, but nearly half feel they’re receiving moderate or no support.
Why it’s important: AI's rapid technological progress in the past two years is stunning. While some see it as a risk to humanity, what if we focused more on what could go right? Leaders may increasingly realize we're poised for a new era of productivity, innovation, and progress. The real risk? Thinking too small.
Full report here. Date published: January 2025
ADDITIONAL REPORTS
ADDITIONAL MUST-READS
Agentic AI (19 reports)
Enterprise AI case studies (20 reports)
Playbooks for AI leaders (16 reports)
Enterprise AI market (8 reports)
LEVEL UP WITH GENERATIVE AI ENTERPRISE
Generative AI is evolving rapidly in the enterprise, driving a new era of transformation through agentic applications.
Twice a week, we review hundreds of the latest generative and agentic AI best practices, case studies, and innovation insights to bring you the top 1%...
Explore sample editions:
All the best,

Lewis Walker
Found this valuable? Share with a colleague.
Received this email from someone else? Sign up here.
Let's connect on LinkedIn.