- Generative AI Enterprise
- Posts
- 20 must-read AI case studies for enterprise leaders
20 must-read AI case studies for enterprise leaders
Plus, key takeaways to help you level up fast.
WELCOME, EXECUTIVES AND PROFESSIONALS.
In recent months, hundreds of new enterprise AI case studies have emerged, spanning generative AI, agents, discovery, implementation, infrastructure, and operations.
I analyzed 272 of them (so you don’t have to). Many lack substance.
But these offer measurable results, in-depth solution breakdowns, and innovative, detailed approaches.
Here are the must-reads:
MCKINSEY

Image source: McKinsey & Company
Brief: McKinsey’s case study reveals how it transformed work with its gen AI platform, Lilli. The objective was to build a platform powered by its proprietary knowledge to accelerate and improve insights for its teams and clients.
Breakdown:
Proof of Concept (March 2023, 1 week): A small team built a lean prototype in 1 week and secured investment approval.
Roadmap & Operating Model (April 2023, 2 weeks): Aligned on priority use cases based on value, impact, feasibility and requirements. Set up cross-functional agile squads for delivery.
Development Decisions (May 2023, 2 weeks): Guided by a five-point framework that evaluated cost, scalability, performance, security, and timing. Combined a hyperscaler’s prebuilt model with five of its own smaller expert models for enhanced answer relevance.
Build, Test & Iterate (May 2023, build: 5 weeks, test: 3 weeks): Alpha tested with 200 users, with rapid feedback improving response quality.
Firmwide Rollout (July 2023, 3 months): Gradual rollout over 3 months, available to all employees by October 2023.
The platform helps employees quickly learn new topics, access McKinsey frameworks, analyze data, develop presentations in McKinsey's style, create project plan drafts, and more.
72% of employees are active on the platform, saving up to 30% of time and processing over 500,000 prompts monthly.
Why it’s important: McKinsey's swift development of Lilli demonstrates how enterprises can leverage GenAI to accelerate knowledge work and enhance productivity. McKinsey's full case study also details lessons learned and further insights into the platform.
Full case study here.
META

Image source: Meta
Brief: Meta shared how Aitomatic, which transforms industrial expertise into AI agents, built a Llama-powered Domain-Expert Agent (DXA) to provide expert guidance to field engineers at an integrated circuit (IC) manufacturer.
Breakdown:
The IC producer faced support issues as field engineers struggled to access specialized knowledge, causing delays and inconsistent service.
Aitomatic built a Llama 3.1 70B-powered DXA to capture and scale expert knowledge. Llama was chosen for its customizability and versatility.
DXA development involved capturing expert knowledge, augmenting with synthetic data to expand scenario coverage, and deployment.
The firm anticipates 3x faster issue resolution and a 75% first-attempt success rate, up from 15–20%, with its newly deployed DXA.
With the DXA's efficacy, Aitomatic aims to enable the development of further DXAs, potentially automating the IC design process itself.
Why it’s important: Field engineers now handle customer inquiries with greater speed and independence from senior staff. Using open-source Llama, the IC design firm retains full ownership of its DXA, trained on sensitive, company-specific knowledge, eliminating dependency on proprietary AI models.
Full report here.
MCKINSEY

Image source: McKinsey & Company
Brief: Deutsche Telekom partnered with McKinsey to develop a gen AI-powered learning and coaching engine, helping to upskill 8,000 human agents in the field and call centers to better meet customer needs.
Breakdown:
Deutsche Telekom saw that traditional learning programs were resulting in substantial variation in performance across agents.
They sought to shift from reliance on individual coaching to an AI engine that would power hyper-personalized learning at scale.
The team spent six weeks diagnosing agent needs with millions of data points, then four months building, testing, and refining the MVP solution.
It’s built into agent workflows. For example, if an agent struggles with eSIM activation, they’re prompted to watch a quick training video.
Operational efficiency has improved, and the likelihood of customers recommending the company has increased by 14%.
Why it’s important: Deutsche Telekom demonstrates how enterprises can quickly evolve to deliver scalable, efficient outcomes with AI. Deutsche Telekom SVP Peter Meier van Esch said, “The impact of this work has been profound,” with employees now better equipped to serve customers.
Full report here.
ACCENTURE

Image source: Accenture / MIT
Brief: Accenture, in partnership with MIT, developed a tool to help clients redesign their workforces for generative AI. By analyzing data on tasks, skills, and job transitions, the tool offers insights into AI's impact and effective reskilling strategies.
Breakdown:
While 97% of CxOs believe GenAI will transform their company, only 5% of organizations are actively reskilling their workforce at scale.
The tool enables clients to experiment with simulation models, exploring various scenarios and comparing outcomes for better decision-making.
Adjustable parameters include AI adoption propensity, investment rate, and AI innovation speed, capturing when and how companies invest in AI.
Users can set simulation duration and upload a CSV file for around 70 parameters, or input requirements via a LLM-enabled chat interface.
Simulation results highlight job changes, task shifts, and skills needed, highlighting the importance of reskilling while tracking revenue growth and headcount shifts.
A results summary is also presented through a multi-model approach, transforming visuals into critical insights. Check out the infographic and demo.
Why it’s important: As gen AI starts to disrupt industries, enterprises should understand its impact on workforce dynamics to remain competitive. Accenture’s tool helps make decisions on reskilling and productivity optimization, enabling businesses to adapt and integrate GenAI for improved performance and growth.
Full case study here.

Image source: LinkedIn
Brief: LinkedIn detailed how it built its AI Hiring Assistant with EON (Economic Opportunity Network), a set of custom models that improve candidate-role matching accuracy and efficiency.
Breakdown:
EON's custom Meta Llama models were trained on 200M tokens from LinkedIn's Economic Graph, including member and company data.
Reinforcement Learning with Human Feedback (RLHF) and Direct Preference Optimization (DPO) techniques were used for safety alignment.
LinkedIn adapted foundation models like Llama and Mistral, evaluating them on open-source and LinkedIn benchmarks (see image above).
LinkedIn found EON-8B (based on Llama 3.1) to be 75x cheaper than GPT-4, 6x cheaper than GPT-4o, and 30% more accurate than Llama-3.
LinkedIn is now enhancing its EON models with planning and reasoning capabilities to enable more personalized, agentic interactions.
Why it’s important: To capture ROI, enterprises are increasingly exploring the cost and customization benefits of open-source models. LinkedIn's EON showcases how in-house gen AI innovation with domain-adapted foundation models can improve the recruiter-candidate experience while reducing costs.
Full case study here.
MCKINSEY

Image source: McKinsey & Company / MIT
Brief: McKinsey traditionally relied on manual processes to curate and tag documents in its internal knowledge repository, achieving ~50% accuracy. A new generative AI tool now labels 26,000 documents annually, improving accuracy and efficiency.
Breakdown:
McKinsey’s manual tagging system took 20 seconds per document with ~50% accuracy.
The GenAI tool uses zero-shot classification with GPT, reducing classification time to 3.6 seconds per document and improving accuracy to 79.8%.
The new system saves up to 676 hours of manual work per analyst each year.
Annually, 26,000 documents are automatically labeled, which will enhance metadata for 140,000 weekly user queries in its colleague GenAI chatbot, Lilli.
The solution improves the search algorithm’s performance by improving search relevance and reducing errors.
Why it’s important: Automating document classification enhances efficiency and accuracy while improving McKinsey's knowledge management and responsiveness to client needs.
Full case study here.
UBER
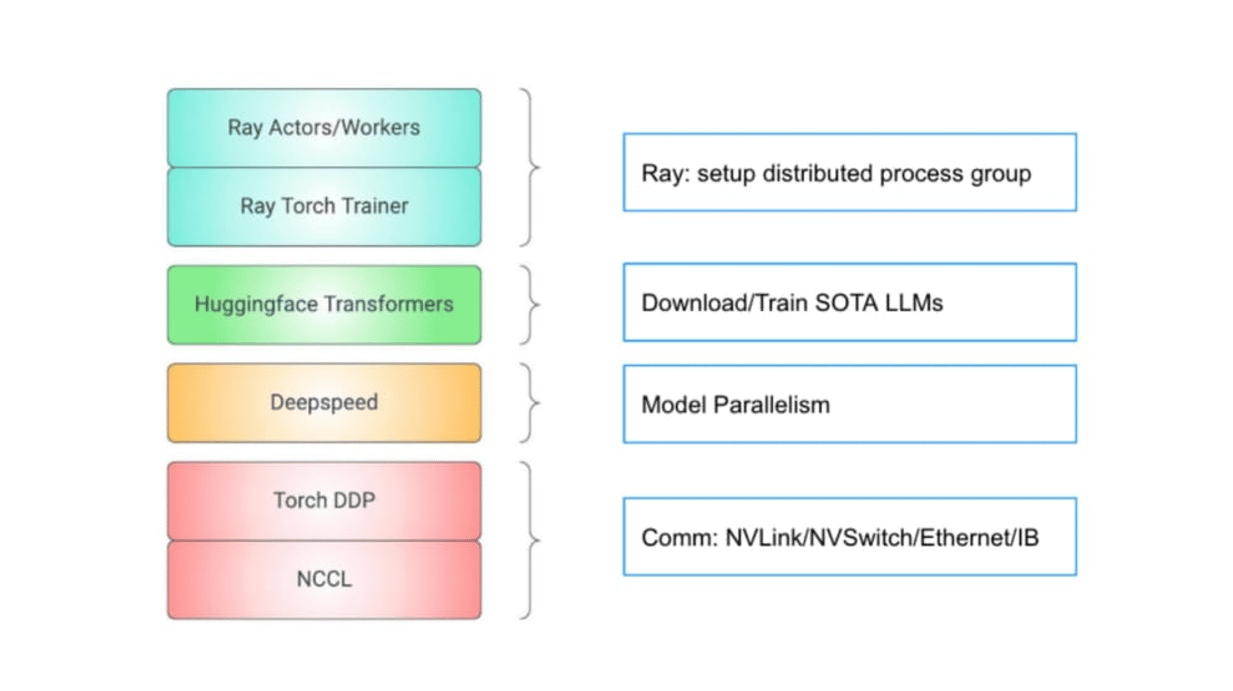
Image source: Uber
Brief: Uber's latest case study, featuring over 2,000 words and high-level architecture diagrams, explores how its in-house LLM training enhances flexibility, speed, and efficiency, using open-source models to help power generative AI-driven services.
Breakdown:
Uber leverages LLMs for Uber Eats recommendations, search, customer support chatbots, coding, and SQL query generation.
The case study details Uber’s infrastructure, training pipeline, and integration of open-source tools, libraries, and models to enhance LLM training.
Uber’s platform supports training the largest open source LLMs including full and parameter-efficient fine-tuning with LoRA and QLoRA.
Uber’s optimizations include CPU Offload and Flash Attention to achieve a 2-3x increase in throughput and a 50% reduction in memory usage.
Open-source models like Falcon, Llama, and Mixtral, along with tools like Hugging Face Transformers and DeepSpeed, enable Uber to adapt quickly.
Uber’s Ray and Kubernetes stack allows rapid integration of new open-source solutions, allowing for faster implementation.
Why it’s important: Uber demonstrates how open-source innovation, combined with robust infrastructure, can improve LLM training, offering a model to help other enterprises accelerate generative AI development and deployment.
Full case study here.
AIRBNB

Image source: Airbnb
Brief: Airbnb's case study highlights the evolution of its Automation Platform, moving from Version 1 with static workflows for conversational systems to Version 2, which supports large language model (LLM) applications.
Breakdown:
The initial platform version supported traditional conversational AI products but faced challenges including limited flexibility and scalability issues.
Experiments showed that LLM-powered conversations provide a more natural and intelligent user experience than more rules-based workflows, enabling open-ended dialogues and better understanding of nuanced queries.
Despite benefits, LLM applications are still evolving for production, such as reducing latency and minimizing hallucinations. These limitations affect their suitability for some large-scale, high-stakes scenarios involving millions of Airbnb customers.
For sensitive processes like claims processing that need strict data validation, traditional workflows are considered more reliable than LLMs.
Airbnb combines LLMs with traditional workflows to leverage the strengths of both approaches and enhance overall performance.
The upgraded platform includes capabilities to facilitate LLM application development, featuring capabilities such as chain of thought, context management, guardrails and observability.
Why it’s important: Airbnb's Automation Platform evolution demonstrates the benefits of merging more traditional rules-based workflows with LLM technology to improve user experience and operational efficiency.
Full case study here.
BOSTON CONSULTING GROUP

Image source: Boston Consulting Group
Brief: A 27-slide publication from BCG offers 42 examples and nine deep drives on how leading enterprises (those scaling AI, per BCG's Build for the Future 2024 Global Study) are generating value with AI, including GenAI.
Breakdown:
The publication showcases 42 examples with measurable outcomes across nine functions: sales, customer service, pricing & revenue management, marketing, manufacturing, field forces, R&D, technology, and business operations.
For instance, a biopharma company used AI in R&D to accelerate drug discovery, achieving a 25% cycle time reduction, $25M in cost savings, and $50M–$150M in revenue uplift.
Nine detailed case studies showcase AI (including GenAI) transformation and impact across functions, such as a GenAI Co-pilot for relationship managers at a universal bank (sales).
Additional deep dives highlight AI for BPO call agents (customer service), GenAI for data governance at a payments provider (technology), AI transforming credit processing at a European bank (business operations), and more.
It explores how AI leaders (26% of enterprises successfully scaling AI value) reshape functions rather than merely deploying or inventing, along with other success factors.
Why it’s important: This publication articulates the transformative value of AI across a breadth of functions, offering deep dive examples and measurable outcomes, all presented in a clear, easily digestible format.
Full case study here.
BMW

Image source: Amazon Web Services
Brief: This case study outlines how BMW Group, with BCG and AWS, implemented its 'Offer Analyst' GenAI application to improve procurement efficiency and accuracy by automating offer reviews and comparisons.
Breakdown:
BMW Group's traditional procurement process involved three main steps: document collection, review and preselection, and offer selection, which include challenges like manual effort, risk of errors, and less meaningful work.
The 'Offer Analyst' GenAI application is designed to help enhance the offer evaluation process, with a user-friendly interface and tailored to the needs of procurement experts.
The enhanced process includes RfP document uploads, offer uploads, information extraction, initial analysis (standard criteria) and tailored analysis (ad hoc criteria), download analysis, and interactive analysis (chat with your offer).
Key solution architecture components of the 'Offer Analyst' include frontend/UI, document storage, integration layer, GenAI layer, API layer, and security features, all built on a serverless AWS architecture for scalability and resilience.
BMW benefits from reduced manual proofreading time, improved decision-making, reduced errors through automated compliance checks, and increased employee satisfaction by enabling more engaging work.
Why it’s important: GenAI applications like 'Offer Analyst' are improving procurement, leading to greater operational efficiency, enhanced employee satisfaction, and a more effective procurement process.
Full case study here.
UBER

Image source: Uber
Brief: Uber's case study explores its centralized Toolkit for building, managing, executing, and evaluating prompts across models. It details the prompt engineering lifecycle, architecture, evaluation, and production use cases.
Breakdown:
Uber’s Model Catalog offers descriptions, metrics, and usage guides for models, while the GenAI Playground allows users to test LLM capabilities.
The Prompt Builder automates prompt creation and helps users discover prompting techniques tailored to their specific use cases.
Prompts can be evaluated against datasets using LLM-based or custom code evaluators.
The architecture features a Prompt Template UI/SDK for managing templates and revisions, integrated with APIs like GetAPI and ExecuteAPI to interact with models.
Models and prompts are stored in ETCD and UCS, driving the Offline Generation and Prompt Evaluation Pipelines.
Prompt templates are reviewed before revisions, deployed with tags, and managed via ObjectConfig, Uber’s internal configuration system, for production deployment.
Why it’s important: Uber's toolkit enhances prompt consistency, reusability, and scalability, improving model performance while safeguarding production environments.
Full case study here.
SAMSUNG

Image source: Samsung
Brief: Samsung leverages RAG to enhance Kubernetes troubleshooting on its Samsung Cloud Platform (SCP), combining LLMs with external data for better technical support.
Breakdown:
Analysis of SCP support records showed 68% of Kubernetes container issues were resolved by users themselves using guides.
Kubernetes containers, integrated with Samsung Cloud Platform (SCP) as the Samsung Kubernetes Engine (SKE), offers added convenience but can complicate troubleshooting when issues arise.
Samsung created SKE-GPT, featuring a diagnostic area that checks cluster statuses against rule sets and SCP products, and an analysis area that generates solutions.
SKE-GPT overcomes the LLM limitation of lacking real-time, domain-specific data by using Retrieval-Augmented Generation (RAG) to incorporate external data, improving response accuracy and relevance.
Samsung's full case study explores indexing (document loaders, text splitters, embeddings, vector stores) and retrieval generation techniques.
Why it’s important: Samsung’s use of RAG demonstrates how GenAI can deliver quick, precise solutions tailored to user needs, based on years of SKE technical support knowledge.
Full case study here.
ADDITIONAL CASE STUDIES
Pfizer - Accelerating Drug Development with Gen AI (full case study)
Comcast - Real-Time Call Response with Gen AI & NLP (full case study)
Takeda - Designing Clinical Trials Faster with Gen AI (full case study)
Uber - Journey to Generative AI (full case study)
Pinterest - Building Text-to-SQL with Gen AI (full case study)
Vimeo - Building Video Q&A with RAG (full case study)
Grab - Classifying Data with Gen AI (full case study)
L’Oreal - Launching GenAI as a Service in 3 Months (full case study)
Amazon - Transforming Java Upgrades with Gen AI (full case study)
Discord - Developing Rapidly with Gen AI (full case study)
ADDITIONAL MUST-READS
Agentic AI (19 reports)
Playbooks for AI leaders (16 reports)
Enterprise AI market (10 reports)
LEVEL UP WITH GENERATIVE AI ENTERPRISE
Generative AI is evolving rapidly in the enterprise, driving a new era of transformation through agentic applications.
Twice a week, we review hundreds of the latest insights on best practices, case studies, and innovations to bring you the top 1%...
Explore sample editions:
All the best,

Lewis Walker
Found this valuable? Share with a colleague.
Received this email from someone else? Sign up here.
Let's connect on LinkedIn.